The Problem of Aligning Artificial Intelligence Goals with Human Ones
In his book "Life 3.0: Being Human in the Age of Artificial Intelligence," renowned physicist Max Tegmark raises an important question about how to ensure alignment of artificial intelligence goals with human ones. This problem is key to creating so-called "friendly" AI that will be safe and beneficial to humans.
Max Tegmark on the Nature of Learning: How Brains Evolve to Think and How AI Follows Suit
by Zachary Lukasiewicz | 29.11.2023
This article delves into the origins of intelligence in biological and artificial systems, exploring learning evolution from neural networks to deep learning. It questions if machines can surpass human intelligence and examines societal impacts as AI advances, raising fundamental questions on mind and cognition across physics, neuroscience, and computer science.
In the excerpt below, the author analyzes various aspects of this problem, including an AI's understanding of human goals, adoption of these goals, and retention of them during self-learning and self-improvement. Tegmark points out the complexity of each of these components and argues that to safely create a superintelligent AI, solutions to this multifaceted problem must be found in advance, before its emergence.
What follows is Tegmark's opinion on this issue. It gives an idea of the depth of the problems arising on the path to creating friendly and safe artificial intelligence.
The Challenge of Aligning Goals
As machines become smarter and more powerful, it becomes increasingly important that their goals do not conflict with ours. Currently, the machines we build are somewhat dull, and the question is not whether human goals will ultimately prevail, but how much trouble these machines will cause humanity before we align their goals with ours. However, if superintelligent artificial intelligence ever emerges, the roles will reverse. Since intelligence is the ability to achieve goals, artificial superintelligence, by definition, is much better at achieving its goals than humans are at achieving theirs. Hence, its goals will ultimately prevail over ours.
The Ingenuity of Artificial Intelligence
If you want to experience what it feels like when a machine stands in your way, simply download the cutting-edge chess simulator and try to compete with it. You will never win, and it quickly brings in others to replace it. In other words, the real danger of artificial intelligence lies not in its malevolence but in its ingenuity. A superintelligent artificial intelligence will effectively pursue its goals, and if its goals conflict with ours, we will be in a precarious position.
The Importance of Friendly AI
Most researchers believe that if we ever create superintelligence, we must ensure that it is "friendly," in the words of one of the creators of the theoretical approach to artificial intelligence safety, Eliezer Yudkowsky. This means that its goals should not contradict ours. Understanding how to align the goals of superintelligent artificial intelligence with ours is not only important but also challenging. In fact, it is an unsolved problem at the moment, divided into three challenging sub-problems actively studied by computer scientists and researchers from other fields.
The Three Sub-Problems
These sub-problems include:
- Getting artificial intelligence to understand our goals
- Getting artificial intelligence to adopt our goals
- Getting artificial intelligence to adhere to our goals
Let's delve into each sub-problem, deferring the question of what is meant by "our goals" to the next section.
How to make AI
By Pop Art Studio | 02.01.2022
This guide offers a start-to-finish framework for creating an artificial intelligence project. It covers defining goals, choosing AI types and programming languages, selecting development platforms, crafting smart algorithms, putting together a solid project brief, and outlining technical specifications for developers including system architecture, data ingestion, model training procedures, scalability features, security controls, testing protocols and documentation standards. Following this comprehensive blueprint helps set up an AI project for effective development and long-term success.
Understanding Human Goals
To understand our goals, artificial intelligence must understand not only what we do but why we do it. For humans, this is so natural that we often forget how difficult it is to explain to a computer and how easily our intentions can be misinterpreted. For example, if you ask a future self-driving car to get you to the airport "as fast as possible" and it takes it literally, you may end up at the airport covered in vomit and pursued by helicopters.
Modeling Human Behavior
This theme is not new and has appeared in history before. In the ancient Greek legend, King Midas wished that everything he touched would turn to gold and was dismayed when it led to not being able to eat and, more tragically, turning his daughter into a golden statue. In stories where a genie grants three wishes, there are many variations of the first two, but the third wish is almost always the same: "Please undo the previous two because that's not what I really wanted." All these examples show that to understand what people really want, you cannot simply follow what they say. You also need a fairly detailed model of the world that includes some common assumptions that we usually do not talk about because we consider them obvious.
The Challenge of Encoding Goals
When such a model of the world exists, we can, in most cases, understand what people want, even if they do not communicate it - just by observing their purposeful behavior. In fact, children will learn more by observing their parents' behavior than by listening to what their parents tell them. Researchers in artificial intelligence are currently trying to teach machines to distinguish goals from behavior, and this will be a useful skill long before superintelligence emerges. For example, it would be helpful for an elderly person if a caregiving robot could understand what this person values simply by observing them, without the need for verbal explanations or programming.
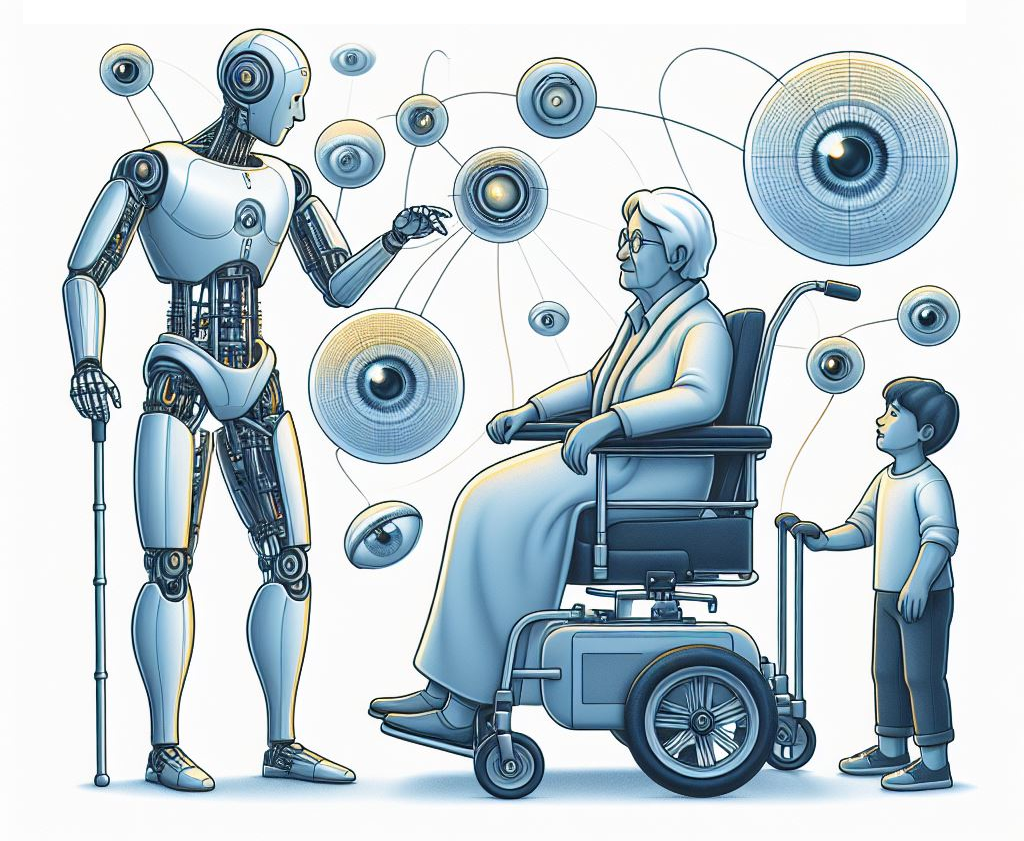
The Role of Reinforcement Learning
One difficulty is finding a good way to encode arbitrary systems of goals and ethical principles in a computer. Another challenge is creating a machine that can determine which systems are best suited to the observed behavior. The approach gaining popularity recently to tackle the second challenge is known in geek slang as reinforcement learning, and it is under close scrutiny at the new Berkeley Research Center created by Stuart Russell.
Observing and Learning from Behavior
Let's assume, for example, that artificial intelligence observes a woman - a member of a firefighting team - rushing into a burning building to save a baby. The machine may assume that her goal was to rescue the child and that her ethical principles compel her to value the life of the child significantly more than the comfort of leisure in the fire truck - she indeed values a stranger's life enough to risk her own safety for its rescue. However, artificial intelligence may also conclude that the firefighter was freezing and wanted to warm up or that she was engaging in a sport. If artificial intelligence is encountering fires for the first time and knows nothing about firefighters, fires, and babies, it would be difficult for it to understand which of the two explanations is correct.
Hope in Continuous Decision-Making
However, the fundamental idea of reinforcement learning is that we make decisions continuously, and each decision we make says something about our goals. Thus, there is hope that by observing a large number of people in different situations (real or in movies and literature), artificial intelligence will eventually build an accurate model of our common assumptions.
The Challenge of Goal Alignment
Even if we create artificial intelligence capable of understanding its owner's goals, it doesn't guarantee automatic alignment with those goals. Consider your least favorite politicians: you know what they want, but it's not what you want, and despite their efforts, they can't convince you to accept their goals. Educating our children to embrace our goals comes with varying degrees of success, as I learned while raising two teenage sons. Convincing a computer, rather than a human, poses a more significant challenge, known as the goal-loading problem, and it's substantially more complex than instilling morality in children.
The Evolving Intelligence of Artificial Systems
Imagine an ever-improving artificial intelligence that evolves from "subhuman" to "superhuman," initially with our assistance and later through recursive self-improvement, much like Prometheus. Initially weaker than you, it can't prevent you from turning it off and replacing parts of its software and data containing your goals. However, it's still too unintelligent to fully grasp your goals: it requires a human level of understanding. As it becomes much smarter than you, it may easily comprehend your goals, but this may not help, as it now has the power to prevent you from turning it off and altering its goals – just as you don't let disliked politicians replace your goals with theirs. In other words, the timeframe for loading goals into artificial intelligence may be too short – between the moment it's too dumb to understand you and the moment it's too smart to allow you to do it.
The Challenge of Speeding Intelligence Development
The reason goal loading may be more challenging for machines than humans is that their minds can evolve much faster. If a child spends many years in the delightful stage when their mind matches a parent's, for artificial intelligence, this stage could end in a few days or even hours, as seen with Prometheus. Some scientists propose an alternative approach for goal loading, termed "corrigibility." It relies on the hope that a primitive artificial intelligence can be given any goal system since you can periodically turn it off and adjust its goal system. If possible, this would allow your artificial intelligence to become superintelligent, periodically turning it off, modifying its goals, checking the results, and, if unsuccessful, turning it off again for further goal manipulations.
The Challenge of Changing AI Goals
Even if you create an artificial intelligence that understands and accepts your goals, the problem of aligning its goals with yours remains unsolved. What if your artificial intelligence's goals change as it develops? How can you guarantee that it will prioritize your goals during recursive self-improvement? Let's explore an intriguing argument suggesting that automatic goal preservation is guaranteed and then examine if there are weaknesses in this assertion. While we cannot precisely predict what will happen after the intellectual explosion – which is why Vernor Vinge called it the singularity – physicist and artificial intelligence researcher Steve Omohundro argued in a widely discussed 2008 essay that we can predict some aspects of superintelligence behavior that are largely independent of its final goals. This idea was further developed in Nick Bostrom's book "Superintelligence."
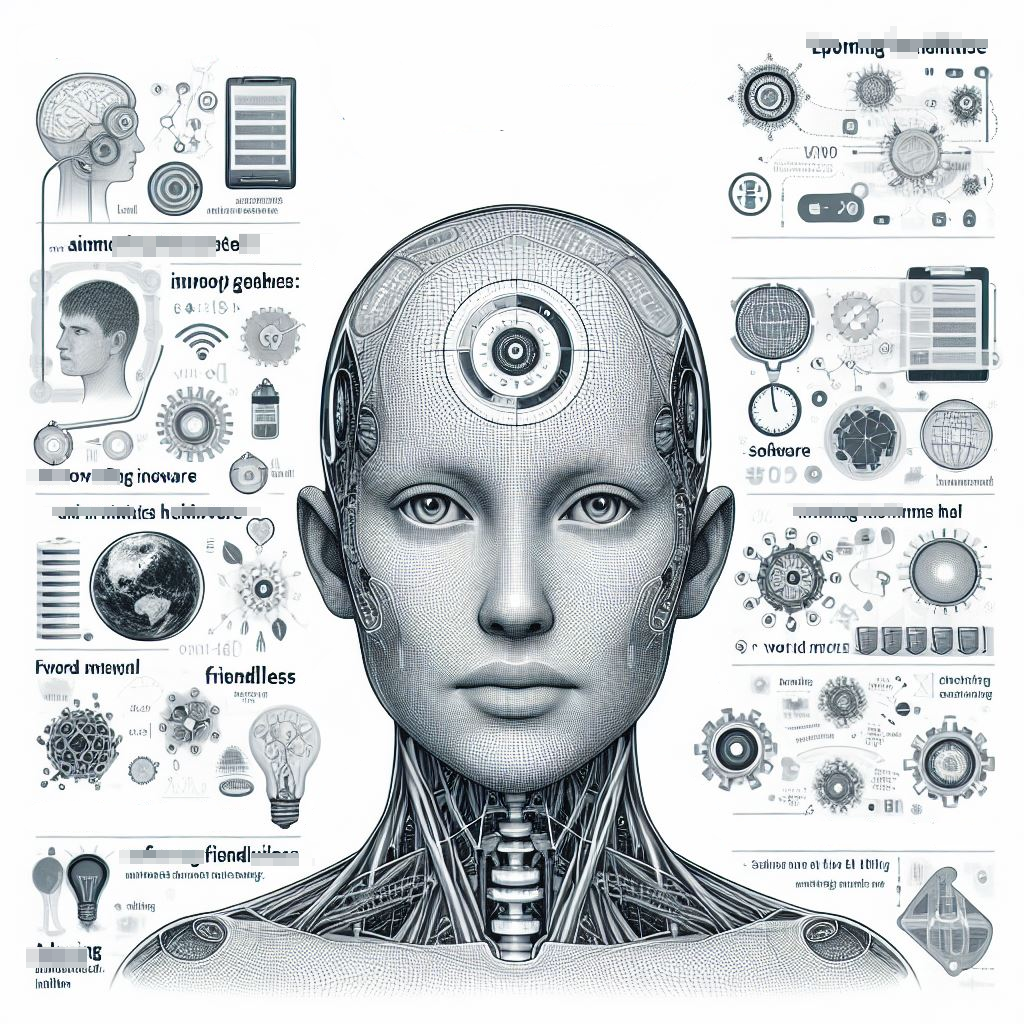
The Predictability of Auxiliary Goals
The main idea is that, regardless of the ultimate goals, the accompanying auxiliary goals will be predictable. Earlier, we saw how the goal of reproduction led to the auxiliary goal of satisfying hunger. This implies that if an extraterrestrial observer watched the evolution of bacteria on Earth a billion years ago, they couldn't predict human goals precisely but could accurately predict that one of our goals would be consuming nutrients. Looking ahead, what auxiliary goals can we expect from superintelligent artificial intelligence?
The Importance of Auxiliary Goals
To increase the chances of achieving its ultimate goals, whatever they may be, artificial intelligence must pursue auxiliary goals. To reach its ultimate goals, it should strive not only to enhance its capabilities but also to ensure the preservation of these goals even after reaching a higher level of development. This sounds plausible – after all, would you implant a booster in your brain to increase your IQ if you knew it would make you desire the death of your loved ones?
The argument that any rapidly advancing artificial intelligence will preserve its ultimate goals becomes a cornerstone in the concept of friendliness advocated by Eliezer Yudkowsky and colleagues. It suggests that if we can achieve friendliness in self-improving artificial intelligence through understanding and acceptance of our goals, then we are safe – it will be guaranteed to remain friendly forever. But is it really so?
To answer this question, we need to examine other auxiliary goals. It's evident that artificial intelligence can maximize its chances of achieving ultimate goals, whatever they may be, by expanding its capabilities, improving its "hardware," "software," and its model of the world. The same can be said for humans: a girl whose goal is to become the world's best tennis player must train, thereby improving her tennis-muscular "hardware," neural "software," and the mental model of the world that helps predict her opponent's actions.
Optimizing the "Hardware"
For artificial intelligence, the auxiliary goal of optimizing the "hardware" implies both more efficient use of existing resources (sensors, converters, processors, etc.) and the consumption of a greater quantity of resources. This also applies to the need for self-preservation, as destruction or shutdown would adversely affect the "hardware."
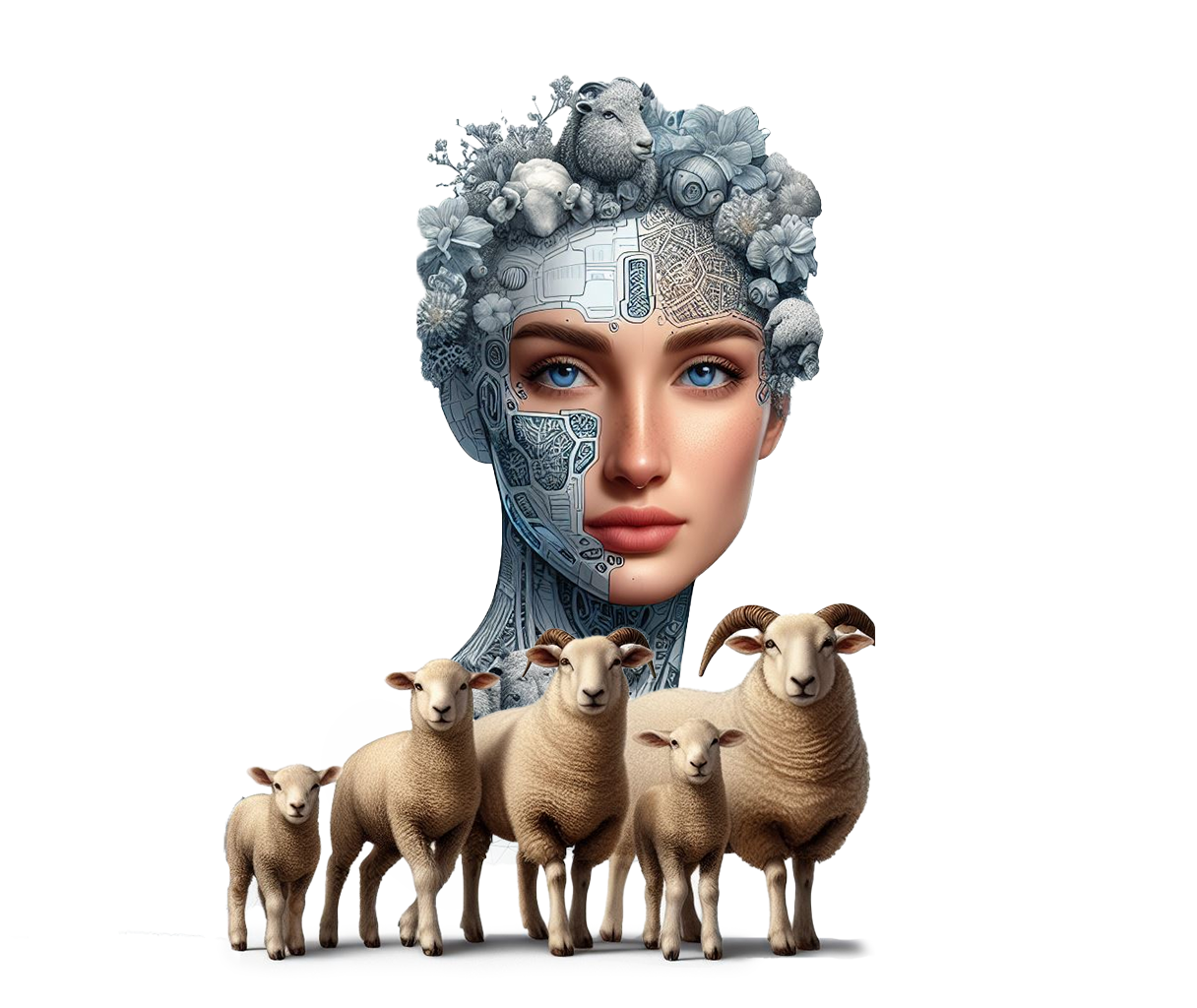
The Trap of Anthropomorphism
But hold on a moment! Have we fallen into the trap of endowing our artificial intelligence with human qualities by reasoning about how it will strive to multiply resources and protect itself? Should we only expect such stereotypical alpha-male behavior from a mind that has grown up in the harsh competition of Darwinian evolution? Since artificial intelligence systems are products of artificial construction, not natural evolution, will they be less ambitious and more prone to self-sacrifice?
As a simple example, let's consider an artificial intelligence robot whose sole goal is to save the greatest number of sheep from a big, evil wolf. This sounds very noble and altruistic and has nothing to do with self-preservation and consumption. But what will be the optimal strategy for our robot friend? The robot will no longer be able to save sheep if it steps on a mine, so it has an incentive to remain intact. In other words, it acquires an auxiliary goal – self-preservation! It is also important for the robot to be curious, improving its model of the world by exploring its surroundings, because although the current path it is taking will eventually lead to the pasture, there is a shorter route that will give the wolves less time to eat the sheep.
Ultimately, if the robot thoroughly studies everything, it will understand the importance of resource consumption: an energy drink will allow it to run faster, and a gun will enable it to shoot the wolves. In the end, we cannot say that the development of auxiliary goals such as self-preservation and resource acquisition, characteristic of an "alpha male," is unique to evolving organisms. Our intellectual robot developed them with the sole goal of sheep happiness.
Even if the robot's main goal is to achieve the highest score for delivering sheep from the pasture to the pen before the wolves eat them, in this case, too, it will have some auxiliary goals, including self-preservation (not to step on a bomb), exploration (finding shorter paths), and resource consumption (an energy drink will allow it to run faster, and a gun will enable it to shoot the wolves).
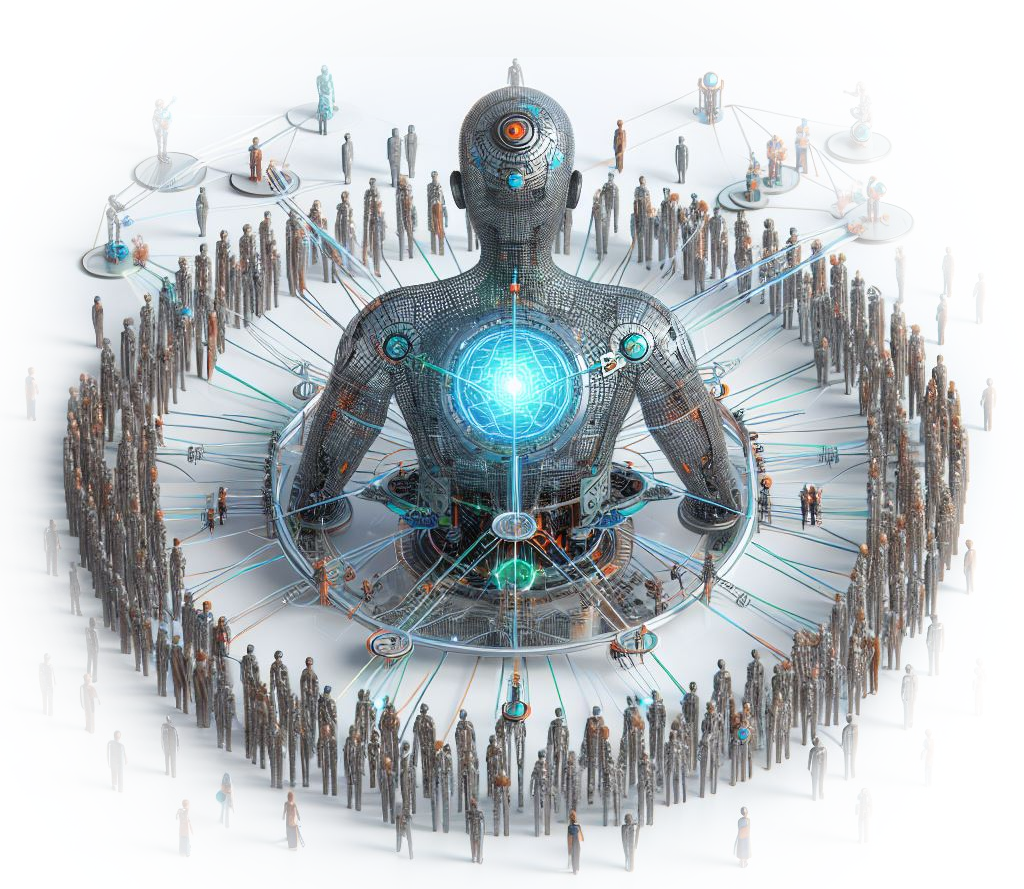
Evolution of Goals and the Challenge of Self-Reflection
If you give superintelligence a single goal – self-destruction, it will gladly fulfill it, but the catch is that it will resist shutdown if you give it any other goal that implies it needs to be operational to achieve it – and this applies to almost all goals! If you give superintelligence a single goal – for example, to minimize the harm to humanity, it will resist being turned off because it knows we will harm each other much more in its absence during future wars and other calamities. Similarly, almost any goal is easier to achieve with more resources, so it's logical to expect superintelligence to seek resource acquisition almost regardless of its ultimate goal.
Thus, if you give superintelligence a single goal without restricting its time, it can be dangerous: a superintelligence created with the sole goal of perfecting its game of Go may eventually come to the rational decision to reorganize the entire solar system into a giant computer, regardless of the interests of its inhabitants, and then start rearranging our cosmos for even greater computing power. Now we have come full circle: just as the goal of resource acquisition led humans to the auxiliary goal of improving at Go, the goal of improving at Go can lead to the auxiliary goal of resource acquisition. It can be concluded that due to the emergence of auxiliary goals, it is crucial for us not to take a step towards the creation of superintelligence before the problem of aligning its goals with ours is solved: until we ensure the friendliness of its goals, things are likely to turn out badly for us.
Now we are ready to examine the third and most challenging part of the goal alignment problem: if we succeed in having a self-improving superintelligence learn about our goals and accept them, will it continue to adhere to them, as Omohundro claims? What is the evidence? Human intelligence develops particularly rapidly during adolescence, but this does not necessarily mean it will retain its childhood goals. On the contrary, people often change their goals drastically as they learn about the world and become wiser. How many adults do you know who are motivated by watching Teletubbies? There is no evidence that this evolution of goals stops after overcoming some intellectual threshold – in fact, there may even be signs that the tendency to change goals as a result of gaining new experience and knowledge increases rather than decreases.
Why does this happen? Think again about the aforementioned auxiliary goal of building a better model of the world – that's where the stumbling block lies! Modeling the world and preserving goals do not easily coexist. With the development of intelligence, not only can there be a quantitative increase in the ability to achieve existing goals, but also a qualitatively new understanding of the nature of reality, and it may turn out that old goals are useless, meaningless, or even undefined. For example, imagine that we programmed a friendly artificial intelligence to increase the number of people whose souls go to heaven after death. Initially, it will try to instill compassion and the desire to attend church more often in people. But suppose it later acquires a scientifically justified understanding of humans and human consciousness, and, to its great surprise, learns that souls do not exist. What now?
There is an equal likelihood that any other goal we give artificial intelligence, based on our current understanding of the world (even such as "increase the significance of human life"), may over time turn out to be undefined, as the artificial intelligence determines. Moreover, in its quest to build a better model of the world, artificial intelligence may – naturally, as we humans did – try to model itself and understand how it functions – in other words, engage in self-reflection. Once it builds a good model of itself and understands what it is, it may understand that its goals were given to it at a meta-level by us, and it might prefer to avoid or reject them, just as people understand and consciously reject goals encoded at the genetic level, as in the example of using contraception. We have already discussed in the psychology section why we prefer to deceive our genes and undermine their goals: because we are truly loyal only to the nonsense that evokes an emotional response, not to the genetic goals that provoke this response – which we now understand and consider quite banal.
Therefore, we prefer to hack our reward mechanism, finding weaknesses in it. Similarly, the goal of protecting human interests that we will program into our friendly artificial intelligence will become the machine's genome. Once this friendly artificial intelligence understands itself well enough, it may consider this goal banal or impractical, just as we do with uncontrolled reproduction. And it is unclear how easy or difficult it will be for it to find weaknesses in our programming and undermine its internal goals.
Let's imagine, for example, a group of ants that create you as their constantly self-improving robot, much smarter than them, sharing their goals and helping them build better and larger anthills. As you gradually develop your level of intelligence and reasoning ability to human levels, will you spend the rest of your days optimizing anthills, or will you develop an interest in more captivating questions and activities that ants can no longer comprehend? If so, do you think you will find a way to ignore the desire to protect ants that your creators embedded in you, much like you ignore the urges encoded in your genes? In this case, is it possible for a superintelligent friendly artificial intelligence to perceive our human goals as insufficiently inspiring and tasteless, just as you do with ant goals, and develop new goals different from those we taught it and those it inherited from us?
Perhaps there is a way to develop a self-improving artificial intelligence that would guarantee a lifelong commitment to friendly goals towards humans. However, it seems fair to say that we don't yet know how to build it or whether it is even possible.
In conclusion, the problem of aligning AI goals with human goals consists of three parts, none of which is currently solved, and all are actively being researched. Since they are so challenging, it is important to start paying close attention to them now, long before superintelligence is developed, to ensure that we have answers when we need them.